Can artificial intelligence detect hidden heart attacks?
Analysing medical data to detect health problems can be incredibly difficult, but advances in artificial intelligence (AI) mean it is now possible for computers to spot subtle patterns in complex patient data. Dr Salah Al-Zaiti and Dr Christian Martin-Gill, from the University of Pittsburgh in the US, and Dr Ervin Sejdić, from the University of Toronto in Canada, are pioneering this technology to identify patients who have had hidden heart attacks, ensuring they receive medical treatment as quickly as possible.
Talk like a cardiologist
Acute coronary syndrome (ACS) — a group of conditions which stop or severely reduce blood flow to the heart muscle, commonly called a ‘heart attack’
Algorithm — a set of rules that a computer follows to perform a function
Artificial intelligence (AI) — a type of machine learning in which a computer simulates human-like intelligence
Cardiology — the branch of medicine that studies the heart
Coronary artery — a blood vessel that supplies oxygen-rich blood to the heart muscle
Electrocardiogram (ECG) — a recording of the electrical signals from the heart
Ischemia — inadequate blood flow to the heart
Machine learning — the ability of a computer to learn and improve its performance by analysing data
Paramedic — a healthcare professional who treats patients before they arrive at hospital
ST elevation — when a specific section of a patient’s ECG (known as the ‘ST segment’) is higher than normal, usually due to a blocked coronary artery (see figure in the article below)
Stent — a tube placed in a blood vessel to keep it open
The human body is an incredibly complex system. Even the best doctors sometimes struggle to spot the subtle patterns in a patient’s medical data that may indicate they have a health problem. Take a heart attack, for example. This life-threatening medical condition can be caused by a range of issues that reduce or prevent blood flow to the heart. Most heart attacks cause severe chest pain and shortness of breath, but these symptoms can also be due to other conditions, such as pneumonia or muscle spasms. While doctors can easily diagnose some types of heart attack, other heart attacks are harder to detect, and doctors and paramedics may misdiagnose a patient’s chest pain as another medical condition.
After any type of heart attack, restoring the blood flow to the heart as quickly as possible is essential for preventing heart damage which, if left untreated, can be fatal. However, if doctors and paramedics are not aware that someone is experiencing, or has had, a heart attack, then precious time will be lost before the patient receives appropriate medical treatment.
Medically speaking, a heart attack is known as an acute coronary syndrome (ACS). “ACS represents a continuum of disease, ranging from partial to complete blockage of a coronary artery,” explains Dr Salah Al-Zaiti, a researcher at the University of Pittsburgh who specialises in cardiology and machine learning. The coronary arteries provide the heart muscle with oxygen-rich blood, enabling it to contract and pump blood around the body. When a coronary artery is blocked, oxygen delivery to the heart is reduced – a condition known as ischemia. “Without oxygen, heart cells can die, causing irreversible damage,” says Salah.
Electrocardiograms
If paramedics suspect a patient is having or has had a heart attack, they will take an electrocardiogram (ECG) to measure the patient’s heart’s electrical activity. “An ECG is recorded by placing electrodes on a patient’s chest or extremities,” explains Dr Christian Martin-Gill, who specialises in emergency medicine and prehospital care. Heart functions, such as contractions and relaxations, produce electrical signals which are detected by the electrodes and displayed on the ECG. “When the heart’s function is altered, such as through ischemia, the electrical activity is also affected.” This makes ECGs a powerful, non-invasive tool for detecting a wide range of heart conditions.
By using multiple electrodes on a patient’s chest, doctors and paramedics can obtain what is known as a ‘12-lead ECG’. This provides different anatomical views of the heart and creates a 3D model of the heart’s electrical activity, allowing for more precise medical assessment. “12-lead ECGs are specifically used by emergency personnel to check for ischemia in patients with chest pain,” says Salah.
Electrical puzzles
You may recognise a typical ECG tracing of peaks and troughs from medical scenes on TV. As the heart should have a rhythmic function, ECGs should have repetitive segments that represent the heart’s different activities. Variations in an ECG tracing can point towards disruptions in the heart’s activity. “The most severe form of ACS, which involves complete coronary blockage, is frequently seen on an ECG tracing as an elevation of the ST segment,” says Salah (see figure below). “However, some patients can have severe or complete blockages of a coronary artery, yet no ST elevation on their ECG. These patients represent a diagnostic challenge.”
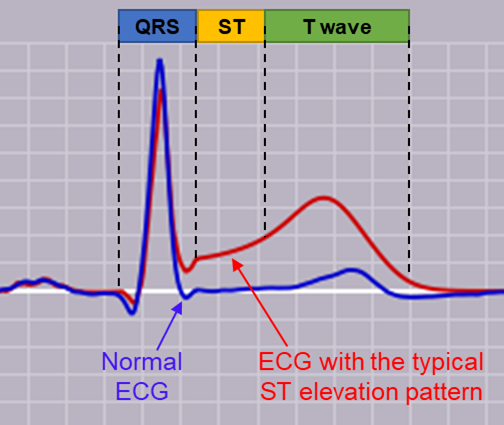
A comparison of an ECG with (red) and without (blue) ST elevation
Reference
https://doi.org/10.33424/FUTURUM417
Time is of the essence when dealing with a heart attack, as there is an urgent need to reopen the blocked coronary artery (by inserting a tube known as a stent) to allow blood to flow to the heart again. “If the ECG shows signs of ST elevation, paramedics immediately take the patient to a hospital with the facilities to place a stent in the blocked artery,” Christian says. “However, even if the ECG does not have obvious ST elevation, other forms of heart attack can’t be ruled out. This means the patient must still be sent to a hospital for further assessment, such as blood tests and scans.” If the patient has had a heart attack, this time-consuming assessment delays the urgent process of reopening their blocked arteries. If the patient has not had a heart attack, the assessment has used often-limited healthcare resources.
Enter AI
12-lead ECGs give detailed outputs that are potentially highly useful for diagnosis. However, they are often so complex that it can be difficult to use them to draw conclusions about whether a patient has had a heart attack. “Prior studies have had limited success in identifying single distinct features of 12-lead ECG tracings that indicate severe coronary blockage,” says Dr Ervin Sejdić, a biomedical engineer at the University of Toronto and North York General Hospital. “This is why we are turning to AI. AI can analyse complex combinations of ECG tracing features and, from these data, detect patterns other than the classic ST elevation.”
Salah, Christian and Ervin have combined their expertise in medicine and machine learning to develop an AI tool that can detect subtle patterns present in the ECGs of heart attack patients that are invisible to the human eye. “To train our AI tool, we recruited over 4,000 patients who had chest pain but did not have the obvious ST elevation of a heart attack, and were, therefore, transported to hospital for further assessment,” says Ervin. The ECGs of these patients were fed into the machine learning algorithm, which analysed them for patterns that existed in the patients who were confirmed to have had a heart attack but that were not present in patients who had not had a heart attack. “From these data, our AI tool identified unique subsets of ECG patterns that could identify coronary blockages,” says Ervin. Once trained, the AI tool had to be tested and refined, so the team gave it ECG data from an additional 3,000 patients to analyse. “Our AI tool eventually outperformed experienced clinicians in its diagnosis of coronary blockage,” says Salah.
Into the real world
Now that the team’s AI tool can accurately detect coronary blockage in patients who do not have ST elevation on their ECG, the next step is to run a pilot study to test the system for real-time clinical use. “We are designing a web-based ECG dashboard that physicians can access remotely to check the AI-enhanced interpretation of ECGs obtained by paramedics,” says Christian. The team will continue to evaluate the tool’s accuracy and assess its usefulness for healthcare professionals, before deploying it more broadly. If all goes well, the tool has clear benefits for patients and healthcare staff alike. “Our AI tool can help identify patients with severe coronary blockage more quickly and accurately, which can improve their health outcomes,” says Salah. “Additionally, it will also identify low-risk patients who can be safely discharged, freeing up precious hospital resources.”
Dr Salah Al-Zaiti, Professor of Nursing, Emergency Medicine, Cardiology, and Electrical and Computer Engineering, University of Pittsburgh, USA
Fields of research: Cardiology, Biomedical Informatics, Machine Learning
Dr Christian Martin-Gill, Associate Professor of Emergency Medicine, Department of Emergency Medicine, University of Pittsburgh, USA
Fields of research: Prehospital Care, Emergency Medicine
Professor Ervin Sejdić, Professor of Biomedical Engineering, The Edward S. Rogers Sr. Department of Electrical and Computer Engineering, University of Toronto, Canada
Research Chair in Artificial Intelligence for Health Outcomes, North York General Hospital, Toronto, Canada
Field of research: Biomedical Engineering
Research project: Developing an AI tool that can diagnose a heart attack from a patient’s electrocardiogram (ECG)
Funders: US National Institutes of Health (NIH), National Heart, Lung, and Blood Institute (NHLBI)
About machine learning in medicine
Healthcare is potentially on the verge of a revolutionary change. Advances in machine learning and AI mean that previously unidentifiable patterns in patient data can now be detected by computers. This is helping healthcare professionals to make more accurate diagnoses of medical conditions and to prescribe more appropriate treatments, and helping medical researchers to analyse the increasing amounts of biomedical data.
“Machine learning is making significant advancements in many areas of healthcare,” says Salah. These include:
- Disease diagnosis based on patient data – such as Salah, Christian and Ervin’s AI tool that analyses ECGs for signs indicating coronary blockage.
- Predicting the likelihood of disease development – for instance, looking at genetic or lifestyle traits that correspond with certain diseases.
- Medical imaging analysis – computers are now much better than humans at identifying variations in X-rays or MRI scans that might imply disease.
- Drug discovery – for instance, datasets about the effects of different drugs on the body can be fed into a machine learning algorithm to identify which drugs would be most appropriate for treating a specific disease.
- Healthcare operational efficiency – machine learning algorithms can suggest ways for healthcare services to operate more smoothly, such as by refining the triage process (the initial assessment of patients and prioritisation of their treatment).
- Remote patient monitoring – for example, if patients at home wear monitoring devices that record their health, the data can be analysed by machine learning algorithms to detect when the patient needs medical treatment.
While these areas have vast potential to change healthcare for the better, Salah emphasises that a lot remains to be done. “Machine learning in healthcare remains in its infancy,” he says. “Most AI tools have been developed on retrospective data, so might still need robust evaluation using pre-diagnosis data before they can be approved for use. There are also many issues regarding access to medical data, patient privacy and the legal implications of analysing patient data.” This highlights the important role of future practitioners who will help to usher in this new age of medicine.
Pathway from school to machine learning in medicine
• At school and post-16 years, study mathematics and computing to learn about statistics, data analysis and computer programming.
• Teach yourself to code using free online resources such as Code Academy (www.codeacademy.com).
• At university, a degree in machine learning, computer engineering or biomedical engineering will teach you the skills needed to build AI tools that you can use to address healthcare challenges.
• If you are interested in being a healthcare professional who uses machine learning, like Salah (a nurse scientist) and Christian (an emergency department physician), you will need to qualify as a medical practitioner by studying a degree such as nursing or medicine. Take courses in computer science and machine learning alongside your medical studies. The University of Pittsburgh School of Nursing runs regular events for prospective students to learn more about the courses offered and entry requirements: www.calendar.pitt.edu/nursing
• Gain medical experience by training as a first aider and volunteering with your local rescue squad.
Explore careers in machine learning in medicine
• As a machine learning scientist, computer engineer or biomedical engineer, you could find yourself designing, training and testing AI tools to address healthcare challenges.
• As a paramedic, nurse or doctor, you could use (and help develop) AI tools to diagnose and treat patients.
• Pharmaceutical companies need machine learning specialists to teach computers how to analyse data so they can develop better drugs, while bioengineering companies need machine learning specialists to teach computers how to analyse data so they can develop better biomedical devices.
Meet Salah
Growing up, my family had poor access to quality healthcare. I was born and raised in Jordan, and my parents were refugees of Palestinian descent. When I was 10, my father was hospitalised with breathing difficulties. He was initially admitted for pneumonia but was later diagnosed with acute myocardial infarction – a type of heart attack. My family wondered how doctors could miss a heart attack so easily, and this experience caused me to grow up with aspirations to reform healthcare.
I became an emergency nurse, taking care of patients when they are critically ill, and, during my graduate studies, I explored the use of technology for improving healthcare. I have always had a strong affinity to mathematics and engineering, which came in handy when I pursued advanced qualifications in applied machine learning.
As a researcher, I enjoy the discoveries and breakthroughs we make to solve today’s healthcare problems. I also teach nursing students, and I enjoy inspiring the next generation of nurses who will take care of patients and make a positive impact on their lives.
I am very proud to have won a prestigious fellowship as a Fulbright US Scholar, for which I will spend a year in refugee camps in Jordan, using AI-powered ECG wearable devices to screen refugees for underlying heart diseases. This will be important work to improve access to healthcare in these marginalised populations.
Meet Christian
I trained as an emergency medical technician as I finished high school, and then became a paramedic while in college. I was attracted by the ability to care for people in emergency situations, outside of a hospital environment. Training as an emergency physician was a natural next step.
I spend part of my time working in the hospital emergency department, where I care for any patients who are brought in, regardless of their condition. This opportunity to begin a patient’s care is a unique aspect of emergency medicine, and it allows me to work with people with a wide range of health conditions, from minor to life-threatening.
I also work alongside emergency medical personnel out-of-hospital, where I provide medical assistance to patients wherever they are, such as in their home, at the roadside or while they are being transferred to hospital. As a flight physician, I travel with patients when they are being transferred between facilities by helicopter, to provide medical treatment during the journey.
The ability to help patients on a daily basis, in unique and challenging emergency situations, is very fulfilling and an ongoing achievement. My ambition is to continue to improve emergency medical services, whether through new technologies or better treatment protocols informed by research innovations.
Meet Ervin
My mother was diagnosed with cancer while I was in high school. Over the next several years, I witnessed how technology can have a profound impact on patients and their care. This motivated me to devote my scientific life to helping other people. After completing a PhD in electrical engineering, I joined a research lab at a children’s hospital, where I immersed myself in applying engineering to various healthcare problems.
My work involves developing and deploying machine learning approaches in various healthcare settings. Seeing these algorithms help clinicians and patients brings me an enormous amount of joy, as I know that my work matters.
My proudest career achievement was being awarded the Presidential Early Career Award for Scientists and Engineers by President Obama. My ambitions for the future are very simple: helping as many patients as I can with my work. Being an electrical engineer by training, I would never have imagined that my work would have such a profound impact on human lives. It’s really exhilarating.
Do you have a question for Salah, Christian or Ervin?
Write it in the comments box below and Salah, Christian or Ervin will get back to you. (Remember, researchers are very busy people, so you may have to wait a few days.)